This article originates from a presentation at the Revenue Marketing Summit in New York, 2022. Catch up on this presentation, and others, using our OnDemand service. For more exclusive content, visit your membership dashboard.
My name is Milan Coleman and I work at LinkedIn, doing testing and optimization programs for LinkedIn ads. Today, I want to talk about my passion: experimentation.
People don't often think of experimentation as exciting, but I think it's a goldmine for marketers and it's super fun. Plus, it gives you the opportunity to learn so much about your customer through quantitative and qualitative research.
In this article, I want to explore a few questions:
- Why experiment, and what can stand in the way?
Hopefully, you already have a culture of experimentation at your company, but you may still find yourself coming up against certain challenges when you're setting up experimentation programs. - How do we define success and get leadership support for our experiments?
Honestly, this is something I've struggled with throughout my entire career.
Attribution’s obviously an issue for marketers, and so is making leadership understand what success looks like from a testing point of view – it can be hard to translate success from a test into a dollar amount. - How can we establish a system for research, iteration, and sharing past wins and losses?
When you have a large data set, it's hard to keep track of what is and isn’t performing well. Plus, a bunch of different teams might be involved, which can lead to duplicate experiments that run into thousands if not millions of dollars.
To avoid this, you want to stay up to date on what other teams are working on and share your research.
Our title, the $60 million tweak comes from Obama’s 2008 presidential campaign. Optimizely, a company that runs A/B tests, was working on the email campaign and landing page, so they ran multivariate tests on the media and the call-to-action (CTA).
These tests revealed some fascinating insights about bias as well as why experimentation is so important.
For one, they tested video versus image. I'm very biased towards video; it tends to get a lot more engagement so I always assume it’ll win, as did the team working on this campaign.
However, in this test, video was the worst-performing variant. If they hadn't run a test, they might have missed out on $60 million of funding. That's why it's important to validate your ideas with your customer to see what resonates with them; often, it's not what you think it’ll be.
As I mentioned, they also tested the CTA. They tried “Sign up” and “Learn more.” This seems like a small change, but it's about evoking an emotional response in your customer and tapping into what they want.
They don't necessarily want to sign up; they just want to learn more, so that was the winning variant. I really love this test because it's so powerful in showing the power of testing things out and seeing how your customer feels.
Why experiment, and what can stand in the way?
Before we get into the how, let’s look at the why. What are the benefits of creating a culture of experimentation?
Firstly, it provides you with direct ongoing quantitative and qualitative data about what your customers desire. With each experiment, you understand your customer more and more.
Secondly, it's really fun to test out new channels, formats, and strategies. Something we're seeing a lot of success with at LinkedIn is running an AI chatbot.
It’s really interesting to see the impact of new channels, especially if you're an early adopter and you start testing before everyone else jumps on the bandwagon.
If you experiment well, you can increase your revenue quite a bit and spark innovation not just with your marketing, but with your product.
At LinkedIn, we're doing a lot of marketing tests and they’re informing our product roadmap. We’re taking our wins to the product team and demonstrating what customers want to see, then aligning on ways we can work together and improve the product.
We’ve seen some of the benefits of testing, now let’s talk about some possible causes of testing fiascos – we also see those quite a bit in the industry.
- Inability to prioritize ideas: I’d say this is the biggest one. It’s great to have everyone bringing their ideas to the table, but if you don't have a way to prioritize them, you’ve got a problem. You can't do everything at once, so you need to understand how to prioritize.
- Non-subject matter experts involved in the project: It’s okay for them to be involved as long as you establish who’s an expert. Everyone having an equal seat at the table isn’t necessarily a good thing if they don't understand, say, certain aspects of design or tech.
- Attribution errors: This keeps me up at night.
- Unreliable datasets: If you're looking at Google Analytics and it's just not lining up with your other metrics, you’ve got a problem.
- KPIs that aren’t tied to business goals: As web marketers, our KPIs are usually about click-through rates or the time spent on a page, but executives might not understand how that translates into dollar amounts.
- Duplicate tests: You need a way to share information so everyone can reference it and not keep doing the same things over and over again. This is so important because it takes a lot of time to set experiments up and they can get really expensive as well.
How do we define success and get leadership support for our experiments?
It’s crucial to create something that not only your customers like but also something that resonates with leadership – you can't move forward if leadership doesn't buy in.
To make this happen, you need a joint effort from both your internal team and your external customer team.
Step one: Determine what success looks like
The first thing that I’d recommend is to determine what success looks like. What’s a meaningful KPI for your business?
At LinkedIn, we've worked really hard to define our Northstar. It's something we had to align on early to make sure we don’t end up chasing the wrong things.
You also want to make sure that your KPIs are understandable. Ideally, you want a way to estimate the revenue impact of your experiments. If you have data scientists in your organization, ask for their support. That’s helped us a lot at LinkedIn.
Don’t forget that every test should have a KPI and a hypothesis. It seems super obvious, but honestly, in a culture of knowledge, people have all these ideas but they often don't have a hypothesis. They lead with emotion when they want to, say, switch up the color on the website.
That’s not enough.
They need to be able to say, “If we change this, I expect this to happen,” and hopefully you’ll have research to back that up before you even start a test.
Step two: Identify your experts
Everyone at your company is likely an expert in something – that's why they're there, so harness those skills.
Experimentation requires so many different skill sets. You don’t just need a conversion optimization manager or a designer; there are so many important people across the business.
If you can do a project management style task early on to identify, for example, your social media expert, your SEO expert, and your UX expert, you’ll be able to build your stakeholder dream team.
This reduces bottlenecks and bias because you don’t have everyone scrambling to give input on things they might not be an expert in. Plus, relying on everyone’s unique expertise is great for your team. It makes people feel productive when they're doing what they're best at.
Here are some examples of some areas where I’ve found it super helpful to have subject matter experts for scaling high-velocity testing:
Analytics
Ideally, you’ll be using some industry standard tools that people already know, for instance, Google Analytics.
But if you’re using something a little more advanced like Adobe Analytics, people might not know as much about it so it’s going to be really helpful to have some analytics experts on board. That way, you won't have to train everyone on a new tool.
UX research
More and more organizations are starting to understand why it’s so important to have a UX researcher and a UX designer when they're running tests.
They’re going to enable you to do your research, create a prototype, and then test the prototype as a qualitative test, as opposed to running a two-week A/B test.
Data scientists, strategists, SEO teams, etc
To make experimentation work, you need a multi-talented team with a diverse range of skill sets. Having these particular roles is really important.
A lot of companies – even larger ones – don't necessarily invest in these skill sets, but it's very difficult to do a scalable test if you don't have these specialisms in place.
Step three: Create a scalable testing system
So how do you create a scalable testing system, and how do you make it high velocity and able to run multiple tests at the same time? Well, once you’ve identified your experts and stakeholders, you want to set up something that looks like this:
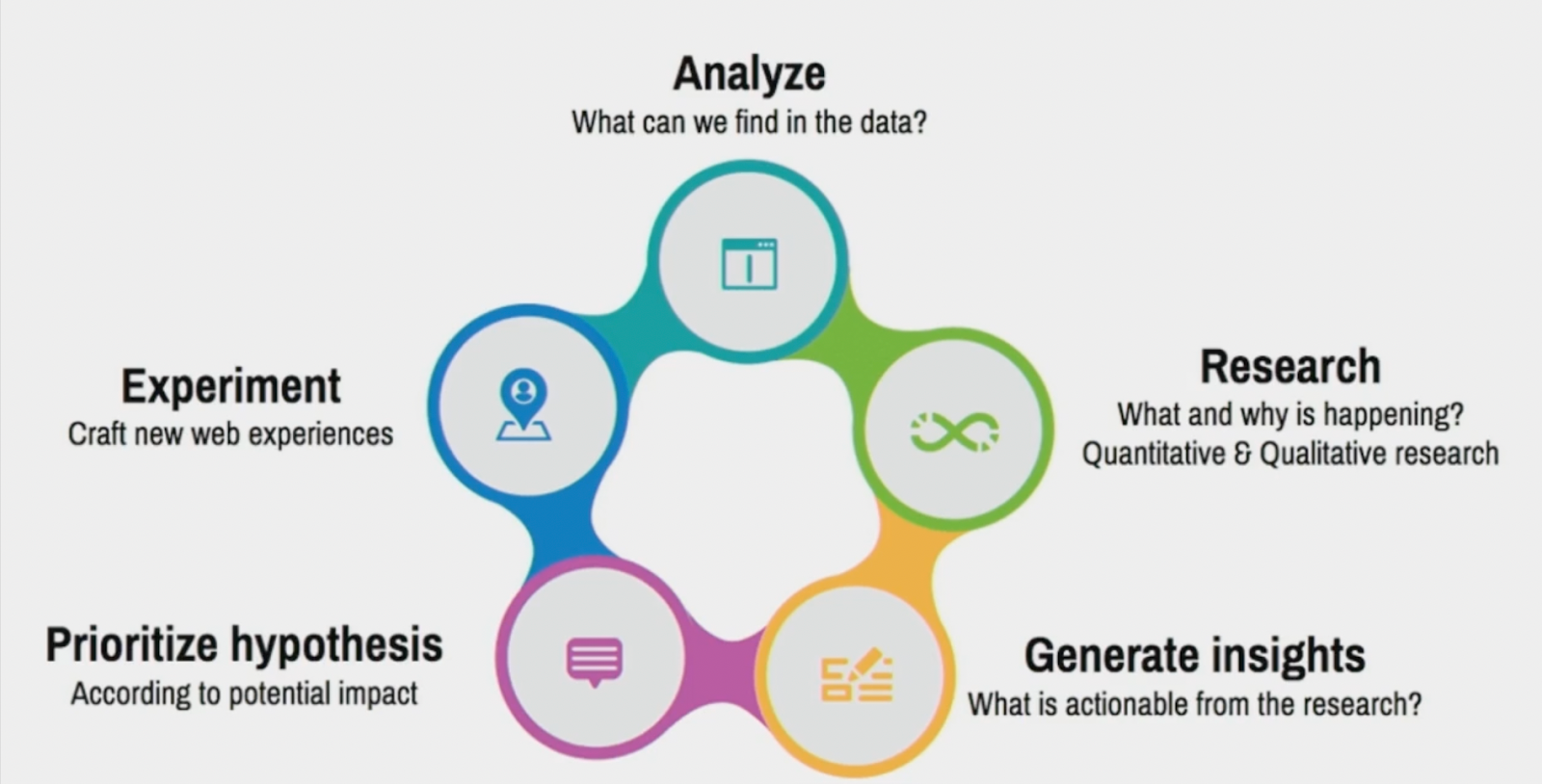
Start by analyzing the data you already have. You want to look for patterns in the pages people are and aren’t engaging with and identify your high-conversion pages, and then you can do some research into those pages.
That's when you’ll start looking at the A/B tests that you've already done and do some qualitative research on why certain things are resonating.
This then becomes a cycle. You look at the insights that you get from your research, and then you can create a hypothesis from it, experiment, and keep iterating.
Throughout this process, as I mentioned, you need to keep focusing on your business goals and hypotheses; while understanding the “what” is great, the “why” is even more valuable.
Step four: Identify your ideal quantitative and qualitative tools
Think about the tools you’re using already. Are they reliable and accurate? Are they easy for you and your team to use? Do they provide helpful and trustworthy insights? Do they do what you need them to do?
For instance, if you’re using a tool for A/B tests but it doesn't let you do multiple tests at once, that might not be the best tool to support your long-term strategy. Look for a tool that you can scale with and grow with and that provides accurate data.
Next, you want to think about which tools are going to help you better understand your customers’ needs. Let’s say you want to know why your customers aren't converting – is there a way that you can put incentivized surveys in place?
The quicker you can iterate the better, so having those systems in place before you even start scaling is really helpful.
Another thing that's been invaluable for us is partnerships with qualitative research agencies.
They’ll help you to deep dive into your ideal customer and find out what's not working in your messaging or your UX, and they can go really deep into every page on your site. They can save you a lot of time with competitor research as well.
Heatmaps are great too. They show you how people are interacting on a page, where they're getting stuck, where they're clicking and not getting a result, what they really want to see, and how far down the page they're going.
That's super exciting for anyone that's into research. I’m a big fan of FullStory because, as the name suggests, it gives you the full story of the customer journey, recording people on the site.
Step five: Get as many ideas as you can and prioritize the good ones
I alluded to this earlier when I talked about what can potentially make your experiment into a fiasco: you need to prioritize the right ideas. It's awesome to get ideas for experiments from other teams, but you’ll have issues if you don't know how to prioritize them.
You have to have an objective framework to assess the business impact of the ideas being put forward so that you know which ones to run with. That’s going to help you take bias out of the picture.
In the past, I’ve used these three quant frameworks:
- PIE framework
- Potential
- Importance
- Ease
- ICE score
- Impact
- Confidence
- Ease
- PXL framework
- Ratings are weighted on what may impact customer behavior
- More objectivity, less guessing
To be honest, they're all pretty similar; they give each idea a value based on a small handful of criteria. Ease is a factor that comes up a lot.
How easy is it to do the test? Do you have to do a whole developer project, or is it something you can roll out in a couple of days? The easier it is, the higher the score.
Impact, confidence, and potential are a little harder to quantify, which is why I prefer the PXL framework. This has literally been life-changing for us at LinkedIn. It gives you a framework based on user behavior and what is most likely to impact it.
For instance, if you land on a site, is the CTA above the fold? If so, it gets a higher score because that's what users see first. Is it something that you’d notice within the first five seconds? That's really important too.
In total, the PXL framework gives you 10 to 15 different ways to rank your proposed tests, which makes life really easy because you can just pick the idea with the highest score.
I've used this for most of the testing projects that I've done because it keeps everything very objective and it's super impactful because you can see the results really quickly.
Organize and share information
To make sure you drive a lot of impact, you need to organize and share your information. This can be tricky, especially at a large company, because you have so much data and it can be hard to keep track of what other teams are working on.
The best recommendation I've gotten is to work with data science. If you have someone on your team that is really good at Looker or Tableau, ask them to build a dashboard for you that shows all the tests that have been running, the wins, and the losses so you can see patterns.
That way, you're not wasting time repeating tests that have already been done. It's easier said than done, but if you can figure out a framework, you’ll save a lot of time, money, and frustration.
Closing thoughts
To close this out, I want to remind you that even if you’re running successful experiments today, there are always ways to improve your processes and grow even faster. Here are a few handy tips to help you do that:
Identify your subject matter experts
It's good to have everyone using their skill set so that they feel confident and you can rely on them. I partner really closely with an SEO expert. It's great for me to be able to ask her questions rather than go in there myself and mess it up.
Meanwhile, I’m bringing my UX expertise to the table, so we can play on each other’s strengths.
Be open to using new tools
There are always new tools on the market that can make your testing strategy easier and more productive. Anything that can automate your processes and personalize your customer interactions is going to be really valuable.
Share your results
Sharing your losses is just as important as sharing your wins because they tell you a lot about if your brand messaging is working or not.
The wins are also super important, and it's a nice little rush of dopamine when you see that what you did worked and you can share that win with your team.